Curriculum Learning for large language models in low-resource languages
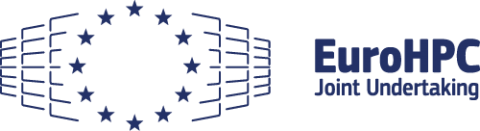

Deskribapen motza, derrigorrezkoa proiektuak logorik ez badu (eu):
Use of computational resources in the EuroHPC SuperComputer to scale up the experiments and build very large models for European languages with few resources
Large language models (LLMs) are at the core of the current AI revolution, and have laid the
groundwork for tremendous advancements in Natural Language Processing. Building LLMs require
huge amounts of data, which is not available for low resource languages. As a result, LLMs shine in
high-resource languages like English, but lag behind in many others, especially in those where
training resources are scarce, including many regional languages in Europe.
The data scarcity problem is usually alleviated by augmenting the training corpora in the target
language with text from a language with many resources (e.g. English). In this project we propose a
systematic study of different strategies to perform this combination in an optimal way, framing the
existing approaches into a more general curriculum learning paradigm. We will use the
computational resources of EuroHPC to perform a systematic study and scale up experiments to
build LLMs for four European languages with few resources. The results of the project will help
fostering NLP applications in these languages, and closing the existing gap between minority
languages and English.
Deskribapen motza, derrigorrezkoa proiektuak logorik ez badu (en):
Use of computational resources in the EuroHPC SuperComputer to scale up the experiments and build very large models for European languages with few resources
Deskribapena (en):
Large language models (LLMs) are at the core of the current AI revolution, and have laid the
groundwork for tremendous advancements in Natural Language Processing. Building LLMs require
huge amounts of data, which is not available for low resource languages. As a result, LLMs shine in
high-resource languages like English, but lag behind in many others, especially in those where
training resources are scarce, including many regional languages in Europe.
The data scarcity problem is usually alleviated by augmenting the training corpora in the target
language with text from a language with many resources (e.g. English). In this project we propose a
systematic study of different strategies to perform this combination in an optimal way, framing the
existing approaches into a more general curriculum learning paradigm. We will use the
computational resources of EuroHPC to perform a systematic study and scale up experiments to
build LLMs for four European languages with few resources. The results of the project will help
fostering NLP applications in these languages, and closing the existing gap between minority
languages and English.
Deskribapen motza, derrigorrezkoa proiektuak logorik ez badu (es):
Use of computational resources in the EuroHPC SuperComputer to scale up the experiments and build very large models for European languages with few resources
Deskribapena (es):
Large language models (LLMs) are at the core of the current AI revolution, and have laid the
groundwork for tremendous advancements in Natural Language Processing. Building LLMs require
huge amounts of data, which is not available for low resource languages. As a result, LLMs shine in
high-resource languages like English, but lag behind in many others, especially in those where
training resources are scarce, including many regional languages in Europe.
The data scarcity problem is usually alleviated by augmenting the training corpora in the target
language with text from a language with many resources (e.g. English). In this project we propose a
systematic study of different strategies to perform this combination in an optimal way, framing the
existing approaches into a more general curriculum learning paradigm. We will use the
computational resources of EuroHPC to perform a systematic study and scale up experiments to
build LLMs for four European languages with few resources. The results of the project will help
fostering NLP applications in these languages, and closing the existing gap between minority
languages and English.
Kode ofiziala:
EHPC-EXT-2024E01-042
Ikertzaile nagusia:
Aitor Soroa
Erakundea:
EuroHPC Joint Undertaking
Saila:
Hitz Zentroa
Hasiera data:
2024/10/10
Bukaera data:
2025/10/09
Taldea:
IXA taldea
Taldeko ikertzaile nagusia:
Aitor Soroa
Ixakideak:
Kontratua:
Ez
Webgunea:
http://